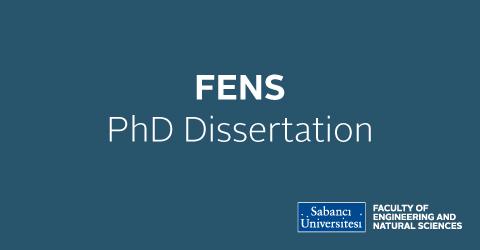
NOVEL GRADIENT-BASED METHODS FOR DATA DISTRIBUTION AND PRIVACY IN DATA SCIENCE
Nurdan Kuru
Industrial Engineering, PhD Dissertation, 2019
Thesis Jury
Prof. Dr. Ş. İlker Birbil (Thesis Advisor), Assist. Prof. Dr. Sinan Yıldırım (Thesis Co-advisor),
Prof. Dr. Kerem Bülbül, Prof. Dr. A. Taylan Cemgil, Assist. Prof. Dr. Mert Gürbüzbalaban, Assoc. Prof. Dr. Özgür Martin
Date & Time: September 20th, 2019 – 20:00
Place: FENS G032
Keywords : large-scale optimization, differential privacy, momentum-based algorithms
Abstract
With an increase in the need of storing data at different locations, designing algorithms that can analyze distributed data is becoming more important. In this thesis, we present several gradient-based algorithms, which are customized for data distribution and privacy. First, we propose a provably convergent, second order incremental and inherently parallel algorithm. The proposed algorithm works with distributed data. By using a local quadratic approximation, we achieve to speed-up the convergence with the help of curvature information. We also illustrate that the parallel implementation of our algorithm performs better than a parallel stochastic gradient descent method to solve a large-scale data science problem. This first algorithm solves the problem of using data that resides at different locations. However, this setting is not necessarily enough for data privacy. To guarantee the privacy of the data, we propose differentially private optimization algorithms in the second part of the thesis. The first one among them is a smoothing approach which is based on using the weighted averages of the history of gradients. This approach helps to decrease the variance of the noise. This reduction in the variance is important for iterative optimization algorithms, since increasing the amount of noise in the algorithm can harm the performance. We also present differentially private versions of a recent multistage accelerated algorithm. These extensions use noise related parameter selection and the proposed stepsizes are proportional to the variance of the noisy gradient. The numerical experiments show that our algorithms show a better performance than some well-known differentially private algorithms.